Please Stop Talking About AGI
Why I think Yann Lecun was right about LLMs (but perhaps only by accident)
It’s become very popular over the last few years to speculate how close society might be to Artificial General Intelligence (AGI). What AGI actually means is murky, and often-debated, but mentioning AGI is usually a good jumping-off point for discussions of future artificial intelligences’ capabilities. Many following the field maintain AGI timelines, rigorous guesses for the probability of this mythical intelligence to emerge at future points. Those in the know might ask you for your timelines over coffee, classifying them as “long” – that it might be a decade or two before AIs are smart enough to take all of our jobs – or “short” – that it could happen any day now.
This isn’t the most useful way of thinking about the progression of AI capabilities. The existence of a timeline implies AGI has a rigorous definition and can be measured. It also implies that AGI is inevitable, the only question being when it will arrive.
What I see is not a march towards complete general intelligence, but rather a trend of increasing AI productivity per unit of human input. This trend holds across many disparate applications. Our AIs can label more data, write more code, do more math, as well as drive cars and pilot planes for longer with less intervention from us. It may be possible that we’ll never reach a point where AIs can run forever, uninterrupted, without human guidance. Rather we’re pushing the boundary of how much we can get for what we give.
Instead of talking about the mythical final frontier of AGI, I think we should start thinking more realistically and measuring the ratio of human input per useful AI output.
Imagine for a moment the curve of how much we input have to provide for a unit of economic value the computer produces, and how this has changed over time. A very rough estimate is pictured above; one important open question is whether we’re approaching some unknowable carrying capacity, or if this figure will eventually decay to zero. (If this happens, it means that computers will be able to produce economic value with zero human input. This would be a frightening outcome.)
To understand what I mean better, let’s take a trip back in time to 2017…
We’ve seen this before (in self-driving cars)
If you’re new to the AI field, you should know that before language models, there was a previous AI craze circa 2017: the rise (and fall?) of the self-driving car.
If you’re not new to AI, let me remind you.
Around that time, several companies declared that within a year they would have Fully Self-Driving cars. Billions of dollars were raised. Millions of miles were driven. Many companies were founded, some of which eventually went bankrupt.
And years later, we’re still not quite at FSD. Teslas certainly can’t drive themselves; Waymos mostly can, within a pre-mapped area, but still have issues and intermittently require human intervention.
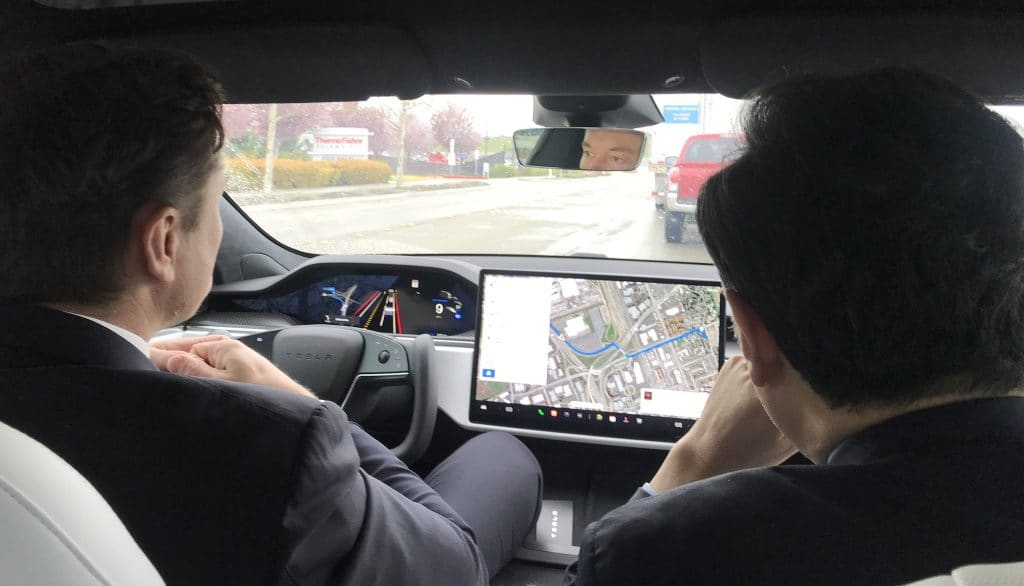
In response, the field has moved on from speculating the exact point cars will be fully-self driving. People instead discuss miles-per-disengagement (or miles-per-human-intervention). How far can the car drive without a human getting involved? This new lens gives us something that we can measure and track over time. Better technology gives us more miles driven per necessary human action.
What does the future look like for FSD? A recent report said Teslas can drive thirteen miles per human intervention; this estimate feels a little low to me, but still seems pretty good. We can certainly drive this number up with bigger models, faster inference, more data, and improved overall engineering.
A crucial question is whether with current technology, the miles-per-intervention number is bounded by some theoretical limit we don’t understand. We don’t know whether our models will keep getting better forever (approaching infinite miles driven with no interventions) or if there really is some amount of human intervention that will always be necessary.
Why Yann Lecun was wrong (kind of)
Now let’s apply this idea to today’s AI craze: language models.
A few years ago, Meta’s Chief AI Scientist Yann Lecun gave a talk about how language models won’t give us a direct path to human-level intelligence. He argued that because language models generate outputs token-by-token, and each token introduces a new probability of error, if we generate outputs that are too long, this per-token error will compound to inevitable failure.
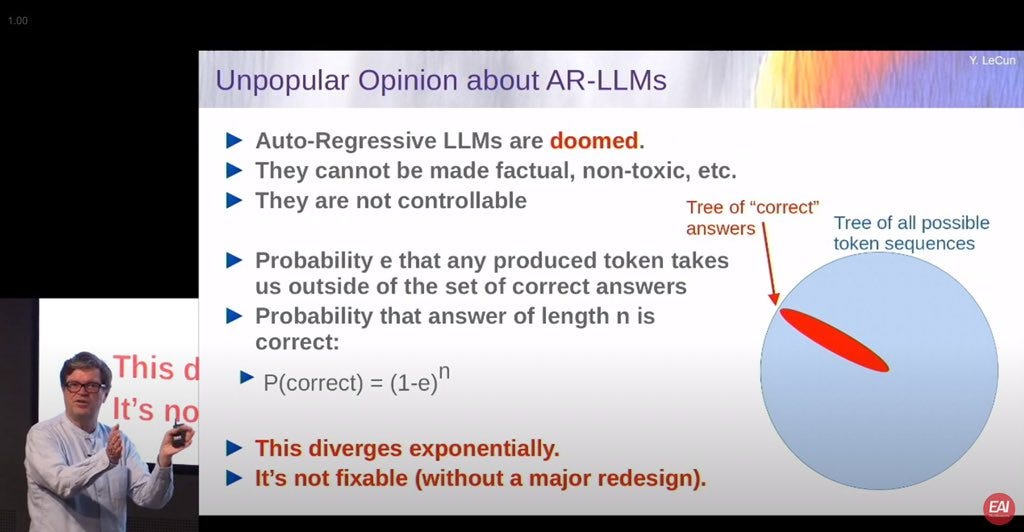
Yann has used this simple argument to explain to the masses why we shouldn’t work on language models if we care about achieving human-level AI. He presents this problem of compounding errors as a critical flaw in language models themselves, something that can’t be overcome without switching away from the current autoregressive paradigm.
But this has turned out to be wrong. A few new AI systems (notably OpenAI o1/o3 line and Deepseek R1) contradict this theory. They are autoregressive language models, but actually get better by generating longer outputs:
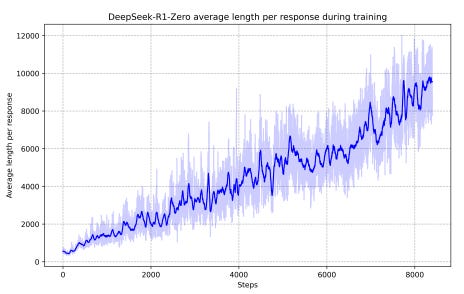
The finding that language models can get better by generating longer outputs directly contradicts Yann’s hypothesis. I think the flaw in his logic comes from the idea that errors must compound per-token. Somehow, even if the model makes a mistake, it is able to correct itself and decrease the sequence-level error rate. This is an incredible development, and was not the case with prior generations of LLMs.
And it turns out that the models’ mechanisms for correcting themselves are interesting and interpretable:
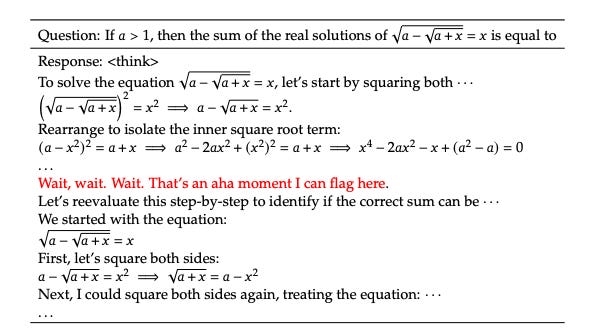
As shown in the image, models can in fact increase their likelihood of success mid-sequence by generating specific strings of tokens. A cottage industry of research is emerging trying to characterize and induce these behaviors, such as “backtracking” to a better solution. (It’s worth noting here that we still don’t know how generalizable these techniques are outside of the types of problems these models were trained on, like coding and math problems.)
Why Yann Lecun was right (kind of)
Naturally, people have been upset about all this. One of the founding members of the field has been giving bad advice to early-stage researchers based on a busted intuition. It’s infuriating, right?
Well, not exactly. I think that people are taking Yann’s argument a little too literally. Yes, we’ve figured out a way to build language models that don’t strictly get worse as we use them to generate longer outputs. But the limiting behavior remains the same: eventually, if we continue generating from a language model, the probability that we get the answer we want still goes to zero.
The practical takeaway from this is that AIs can’t work on their own forever. Lots of people are working on building Agents, systems that use language models to accomplish tasks over long time horizons. But the quest for a fully autonomous agent feels similar to the quest for fully self-driving cars: it might never be possible to build this, at least with the current stack.
There may be a kind of data processing inequality going on behind the scenes. In some sense, the highest-quality information inputted to language models comes from the human-written prompts (and potentially inputs read in via tool use, like checking flight times or the weather). When the language models are left on their own to generate infinitely-long chains of thought, that input “signal” attenuates to nothing; eventually, without further input from a human, those chains of thought lose all meaningful value. Improving our technology can delay this, and improve the quality and amount of work we can do with a single input prompt. But it doesn’t seem likely that I’ll wake up one day next year and this figure (work / prompt) will have spiked to infinity.
This is why measuring language models’ progress in terms of AGI timelines is misguided. We should be thinking about language models the same way we think about cars: How long can a language model operate without needing human intervention to correct errors? Framing our inquiries into language models like this allows us to reconcile Yann’s valid concerns about the models with new advances from OpenAI and DeepSeek—and will also lead to more productive research and conversation in the language model field, as it has with cars.
Instead of waiting for FAA (fully-autonomous agents) we should understand that this is a continuum, and we’re consistently increasing the amount of useful work AIs can do without human intervention. Even if we never push this number to infinity, each increase represents a meaningful improvement in the amount of economic value that language models provide. It might not be AGI, but I’m happy with that.
Not sure I like this framing of Teslas can’t self-drive themselves. If as you say they can self-drive for 13 miles before needing human intervention, then it seems like they can self-drive for 13 miles.
Humans make many forms of driving mistakes all the time. They take wrong turns, they drive in the wrong lane, they speed, they go too slow, they wreck. If another human was overseeing them, they would make fewer mistakes. Maybe they’d go from 1 crash every 1million miles to 1 crash every 10 million miles. But by your definition, because they still need oversight, they cannot fully self drive.
I think this is misguided for a couple of reasons.
First the argument shows a contradiction, but doesn’t really show strong support for it. Its a guess which doesn’t show strong reasoning. We have an example where that seems to be the case, but if we accept the premise as true, it should be true both ways and its not (forward and backward). We have a set of LLMs that don’t improve, and so this segment must be apples to oranges without understanding the difference, and context is important. Paraphrasing can easily lead people astray, where something was said with a distinct meaning that is then taken out of context (overgeneralized), and I think this may have happened here, but I can’t be sure without the links to what was exactly said by Yann Lecun (referenced). I haven’t been able to find a talk with that slide in it, but I don’t have a lot of time to look.
Second, few people seem to realize that all of western society depends upon the value of labor being sufficient to purchase enough goods to support themselves, a wife, and children (so at least 1 has children themselves). This goes to the basis of a distribution of labor. When you have machines capable of replacing most people it all breaks down, and then people starve. This is for a number of reasons.
AI lets you replace people at the entry level, and talent development is a sequential pipeline. No new people in, no new people out. That’s within a 10 year time horizon.
Human’s are notoriously bad at recognizing and seeing failures which are slow moving. Dam breaks, avalanches, money printing (unreserved debt issuance), and the chaotic socialist calculation problem, are just some examples…
We’ve reached the limits of growth, and the birth rate has declined because people are unable to support the second reason here (its no longer true), the old hoarding resources are crowding out the unborn young, and soon legitimate producers will shut down when they can no longer make profit (as happens when stable store of value is lost). Current projections of birth-rate decline show in 8 years, we will have more deaths than births (not factoring in mortality within the first 2 years).
This leaves only state-run apparatus (faux producers) funded by money printing, that create distortion to extract sufficient profit. Distortion prevents economic calculation, and might include artificial supply constraints, price fixing, and other forms of corruption (such as buying back bad meat so no loss leader sales occur, people need meat so they are forced to buy it at higher price), it all leads to that last problem after the market has collapsed to non-market socialism, the socialist calculation problem where shortages sustain and get worse.
Like a limited-visibility n-body problem (modern literature), the intersection of economics and monetary policy in such cases becomes chaotic (Mises), an ever more narrow safe path forward based on lagging indicators, and when it fails exchange fails, then production fails, and Malthus/Catton show this results in famine which causes a great dying, and the sustainable population being less than it was before such improvements previously (<4B people globally). This is a cascading failure.
A three-body problem’s general solution is un-solveable. So the question here really is, should we be focusing all of our investment into models that will ultimately end up destroying us, where the process of integration burns the bridges preventing us from backing out afterwards?
Great men of the past understand that they cannot know the future, and will inevitably be mistaken as a result. In the past they created systems that can be corrected when those things occur, but the generation today seem to have forsaken this sentiment, being more intent on removing agency and its accompanying resiliency, in lieu of fragile mechanisms of coercive control (slavery of the unborn). Thomas Paine called these systems in his work Rights of Man, “dead men ruling”. Its a recurring theme throughout history, and the competition dynamics will force a race to the bottom without any net.